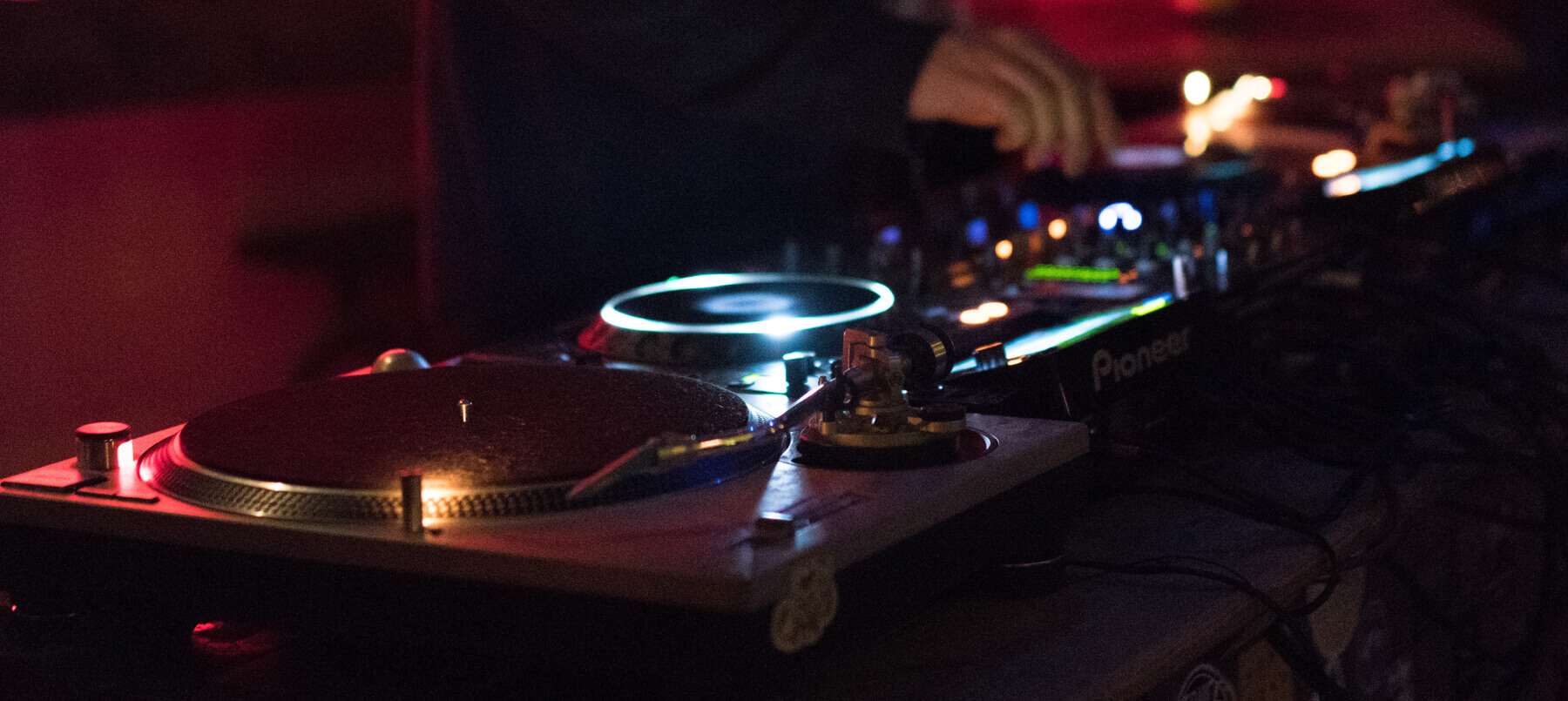
-
Client
The Customer is one of the largest German music production centers. Having been the market leader in this niche for years, the Customer turned to Jelvix to create innovative software for the music industry.
-
Business Challenge
The Customer hired Jelvix to create an algorithm that can detect and extract chords and notes from audio or video input on the fly, with the possibility to synchronize detected chords/records with the source input. The main challenges in developing the project were achieving maximum accuracy in determining chords, the lack of music for tagged source data, and restrictions on development time, memory, and GPU power.
-
Solution
To create a superbly working algorithm for detecting and extracting chords and notes, the Jelvix development team decided to use the supervised methods of Machine Learning. For the platform to perform tasks at a maximum speed and to be able to cope with a broad set of data and receive the desired accuracy, we decided to use a GPU.
- Location
- Germany
- INDUSTRY
- Entertainment
- SERVICES
- ANN model development
- TECHNOLOGIES
- Python 3.7, Tensorflow 1.14.0, Keras 2.3.0, Librosa 0.7, Jams 0.3.4, Crema 0.2.0, Pumpp 0.5.0, Muda 0.3.0, Pescador 2.1.0, Pyrubberband 0.3.0, Harmonic Constant-Q Transform Feature Extraction, From -6 till +6 Semitones Data Augmentation, Deep Learning with advanced callbacks, Mirex points for accuracy evaluation
Product Overview
Client’s goals
The Сustomer decided to develop this technology to bring the music industry to a whole new level. Working with musical compositions, from creating to parsing, always takes a lot of time at any production center. Multilevel and complex work exhausts a lot and leaves neither power nor time for creativity. This prompted the Customer to change the process of working with music tracks. The desire to make all the processes more automated and faster led him with the project idea to the Jelvix team. We focused on creating a flawlessly working product that would fulfill all the Customer's goals.
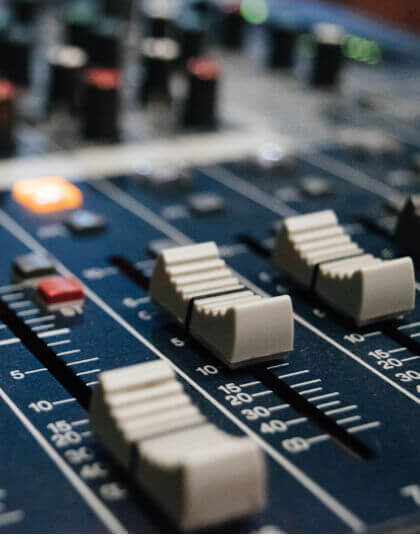
Implementation
The Jelvix project team began their work by collecting all the necessary data. This phase took a lot of time since it was essential to find sufficiently clear and diverse data to avoid the errors in the operation of the technology.
The next step was to study the possible impact on the copyright of the tracks and compositions. There was a variety of checks and tests processing delay degrees using TPU. When the data sets became more substantial, the task execution time exceeded hours, while it should not have taken more than a few minutes. This caused a switch to the GPU to optimize and speed up the data processing.
Further, our development team moved to the effectiveness of the technology. Having determined the technology's desired accuracy as 90%+, an extensive data set was prepared with notes for professional musicians’ checks and tests. The last and final stage was training the neural network and bringing its work processes to reliably accurate.Value Delivered
The Jelvix development team created an innovative technology that has transformed the Customer’s business and brought the music industry's work processes to a completely different level. Having made a massive contribution to the creation and development of the technology, the Jelvix project team built and trained a 9-layer neural network. Its ability to determine chords as quickly and accurately as possible completely satisfied the Customer's business needs.
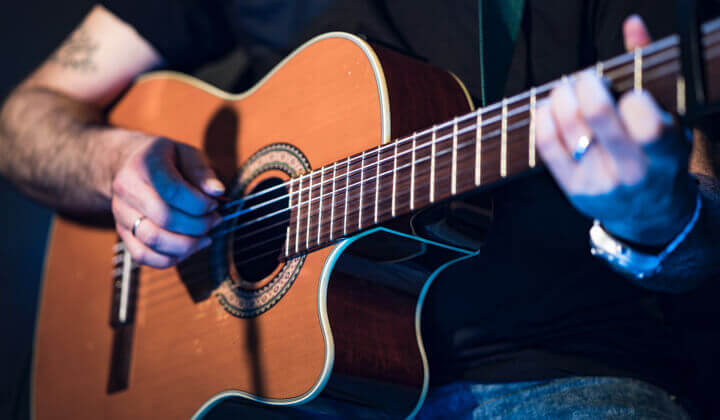
Project Results
The Сustomer received a fully finished, and improved technology on time. As a result, their business became more competitive in the niche by using the ML-trained model, which can detect chords for any song with an accuracy of 85-95%.